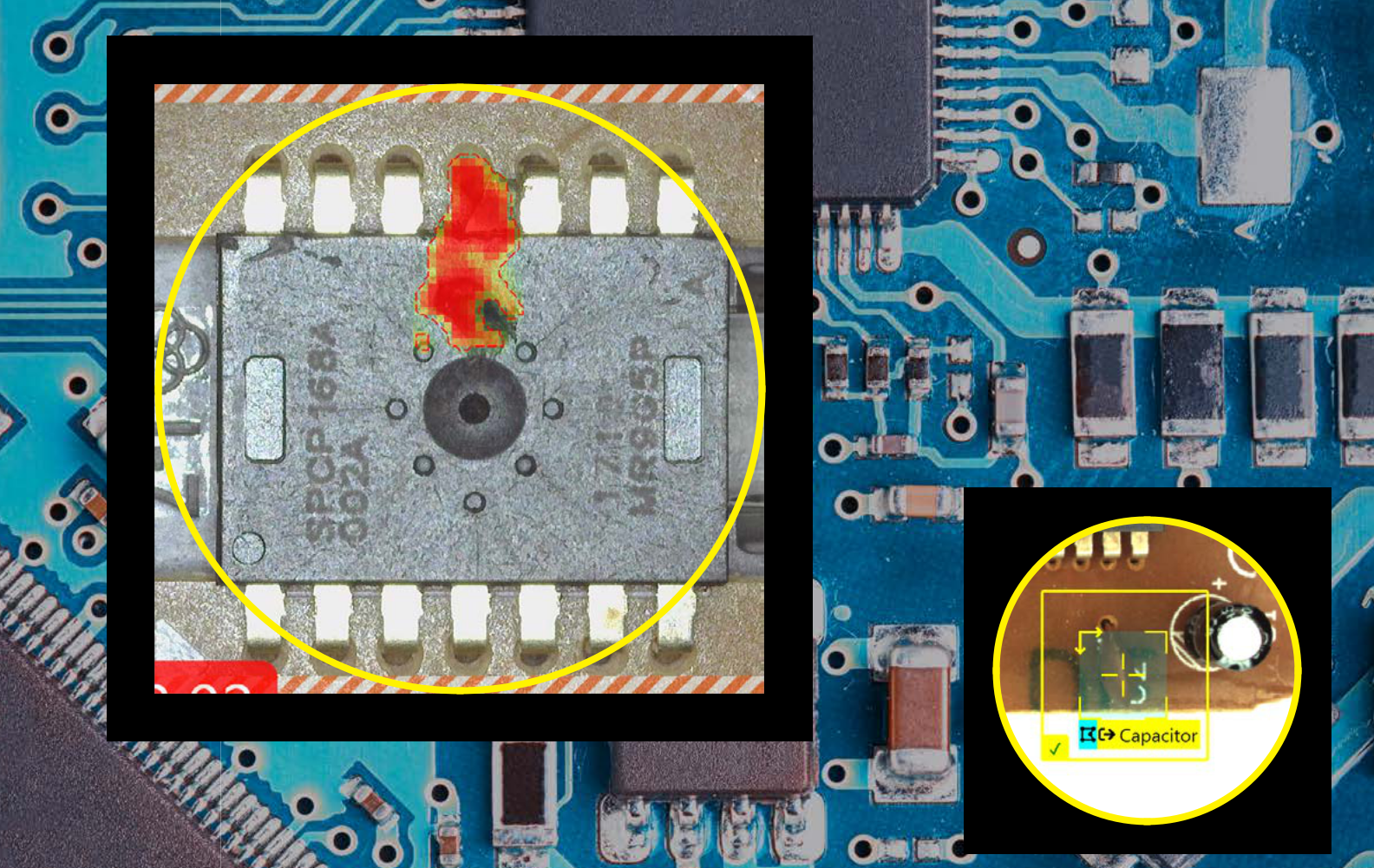
DEEP LEARNING SOLUTIONS IN ELECTRONICS INDUSTRY
Automating the production process and improving quality are two of the biggest needs of the electronics industry. However, some applications are too complex, time consuming, and expensive to program into a rule-based algorithm. Using testers to handle different types of parts and make judgment-based decisions can also lead to errors and slow down production.
Cognex Deep Learning is the first deep learning vision software designed to tackle tough electronics applications that require cosmetic inspection, grading, and character recognition. Cognex Deep Learning provides human-like inspection with the speed and robustness of a computer system, in a field-maintainable unit that makes it easy to train new parts and types. fault in the field.
Precise assembly in electronic board
Problem:
During final assembly verification, 2D and 3D machine vision systems typically inspect the PCB for the presence and correct placement of LEDs, microprocessors, and other surface mount devices. Missing or misplaced components can affect the performance and life of the PCB. These defects must be detected before the PCB is assembled into the device or shipped to the customer. However, small changes in appearance — whether due to subtle light contrasts, changes in viewing angle and direction, or glare on metal surfaces — can confuse an inspection system. automatic. Parts that are close together are difficult for machine vision systems to distinguish as independent parts. Programming these checks into a rule-based algorithm is time consuming, error prone, and challenging for the field engineer to maintain. Test personnel, despite being able to identify these components, cannot meet the demands of high-speed processing.
SOLUTION:
Cognex Deep Learning offers a field-maintainable solution that rivals human inspection for PCB assembly verification. The assembly and part placement verification tool learns to identify components from annotated “good” images, building a reference model of their normal shape. The tool generalizes to the distinguishing characteristics of components based on their size, shape, and surface features, and learns their normal appearance, as well as their general location on the board. At runtime, the tool analyzes all relevant areas of the board to identify and count components, regardless of their variation. This way, the check determines if components are present and can tell if the board has been assembled correctly.
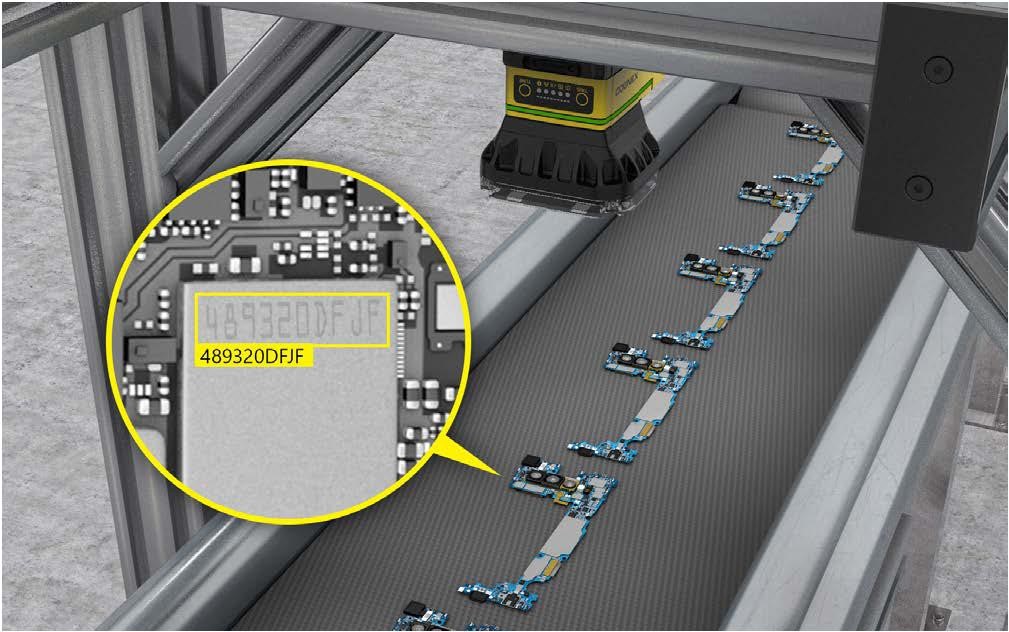
Deep learning to test resistance welds
Problem:
In order for a component like a mouse diode to be mounted without affecting its electrical connection, the solder resistor must be glued cleanly to the bare circuit board. Even minor defects in the solder can cause wire breaks, short-circuits, and other electrical problems. These defects vary in size, shape and appearance due to glare. It is very difficult to program automated tests that can withstand significant part changes under these conditions.
SOLUTION:
Cognex Deep Learning quickly determines solder resistance on a diode when other methods attempt to test under the same lighting conditions. The assembly and part location verification tool trains on a set of representative images of solder resistance to learn the normal morphology of “good” and “bad” solder. During runtime, the tool immobilizes and positions against the PCB, albeit with variation in characteristic luminance. During the second stage of the test, the solder resistor must be checked for any functional anomalies, such as bridging, peaking, or holes. By using the defect detection tool in supervised mode, the user can train the tool on a representative set of known “good” solders and “bad” solders with defects. labeled disability.
Based on these images, Cognex Deep Learning learns the natural texture of the mouse diode, as well as the normal shape of the solder. Additional images can be added to the training set during validation to reflect additional examples and model optimization. Various parameters can be adjusted during the training and validation phase to help explain variations in appearance for accurate detection of all diodes with faulty solder.
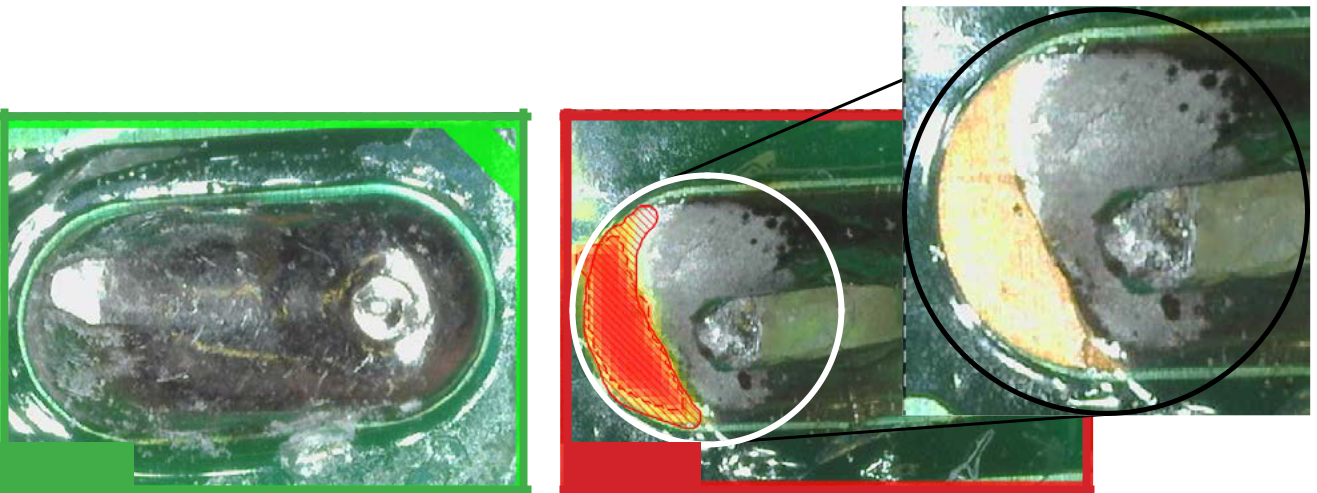
Deep learning checks the aesthetics of the product's exterior
Problem:
Aesthetic inspection can be difficult when parts are different, whether at the component level or at the packaging and case level. Scratches, dents and other cosmetic defects may not affect function but affect finished product quality and consumer perception. Some cosmetic defects may be an obvious cause of rejection, while other minor imperfections may be acceptable. For this reason, manufacturers need to train an inspection system to look for specific defects and distinguish them from minor defects. Programming this complexity check into a rule-based algorithm requires complex error libraries. Human inspection, although more flexible, is too slow, unreliable, and inconsistent.
SOLUTION:
By using the defect detection engine in supervised mode, an engineer can train Cognex Deep Learning to look for specific defects, such as scratches, while ignoring anomalies and variations may not be important. This tool is optimized for working with low-contrast or poorly captured images. For example, the image below illustrates how the defect detection tool analyzes both good and bad images of the earbuds. At runtime, the software described images with severe scratches as defective, having learned to recognize and ignore minor cosmetic blemishes.
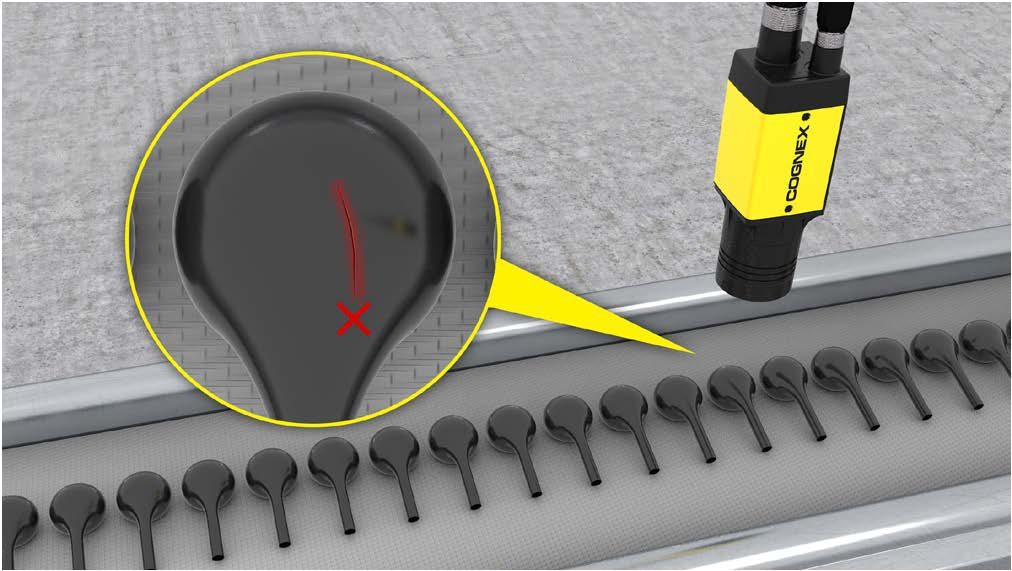
Deep learning checks battery module assembly and aesthetic certification
Problem:
During the pre-assembly insertion test, the phone’s interior is checked for defects before assembling the case. Batteries can be damaged when they are led into the case. Locating and inspecting the battery was difficult as assembling the phone was always difficult and unstable. Deep learning vision software simplifies the automatic detection and characterization of defects in the metal surface of a battery.
SOLUTION:
Cognex Deep Learning allows manufacturers to test battery integrity before a phone undergoes final assembly and to distinguish between aesthetic and functional abnormalities. Using a defect detection tool in supervised mode, an engineer can train the software on “good” images as well as “bad” images with labeled defects. From these images, the tool learns the normal form of the battery, including acceptable natural variations. Parameters can be continuously adjusted during the training and validation phases until the trained model correctly detects and segments all images with functional abnormalities. Once deployed, the fault detection tool will identify and remove the faulty battery.
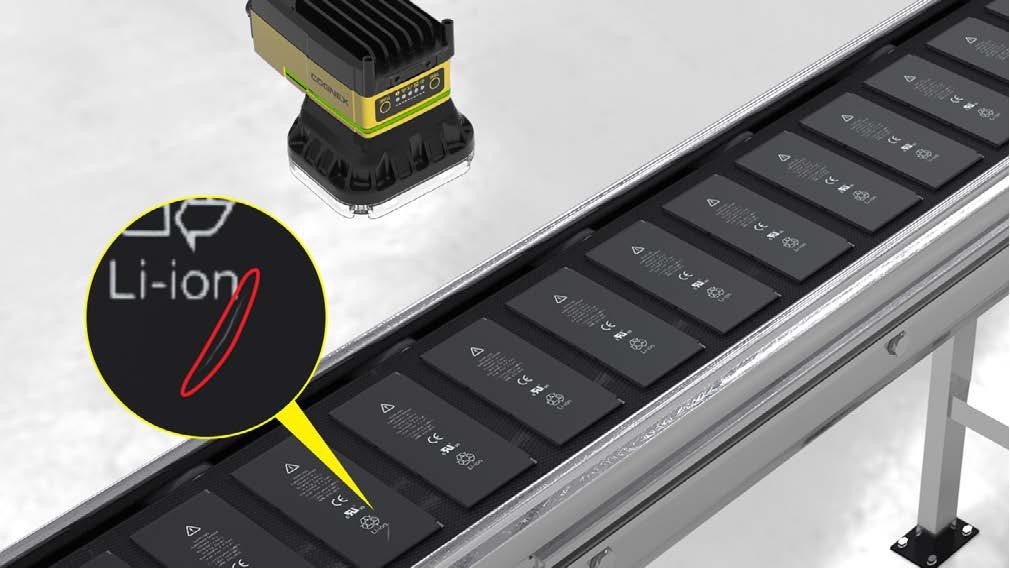
Deep learning aesthetic test IC leads
Problem:
Machine vision is used throughout the semiconductor manufacturing process to closely monitor quality and catch errors. Manufacturers must be wary of pins that are scratched, twisted, bent, or missing. A chip has such low fault tolerance that any defect, even the most superficial, is cause for rejection. With so many types of potential defects, it is not efficient to program the test into a rule-based algorithm. Deep learning vision software can help limit semiconductor defects and improve productivity without using extensive error libraries.
SOLUTION:
Finding all defects explicitly is too complicated and time consuming. Cognex Deep Learning provides a simple solution to identify all anomalous features, even without training on “bad” images. Instead, an engineer uses error detection to train the software on a “good” pattern of images in unsupervised mode. Cognex Deep Learning learns the normal shape and position of the chip’s wires and pins and characterizes all misaligned features as faulty.
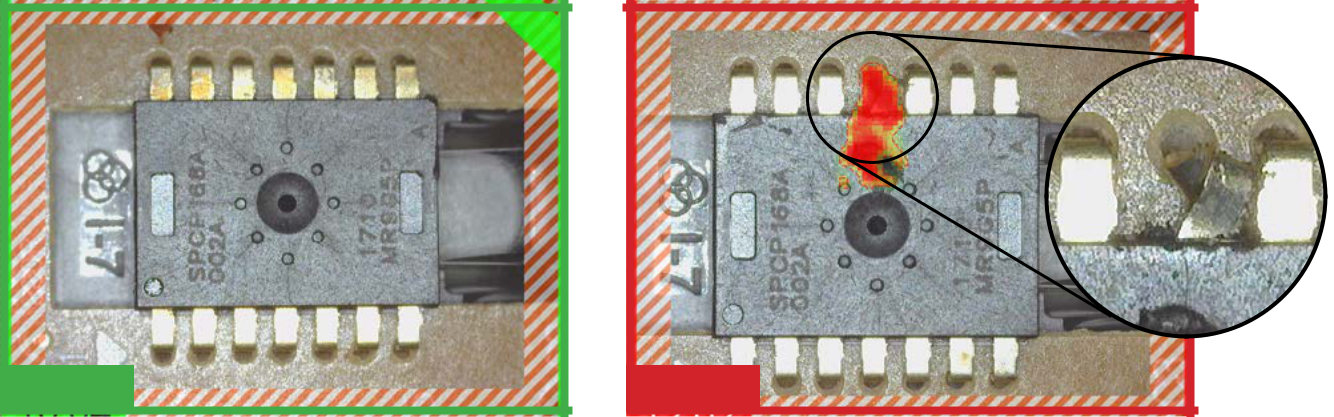
Deep learning capacitor classification
Problem:
Classification of electronic components can be especially difficult when the parts are divided into several layers, each of which has some visual variation. Capacitors vary in type (ceramic and electrical), as well as size and color, depending on their manufacturer and specifications. Even within the same category, there can be confusing variations in the pattern. Their cylindrical shape and lighting can be even more complex. VisionPro ViDi offers a deep learning-based alternative to automate multiple classifications in a single image.
SOLUTION:
Using the fault detection tool, an engineer trains the software in supervised mode on a set of annotated images where both the gold capacitor and the condenser are classified as “good” parts. “. At runtime, the model extracts and segments all capacitors and gold capacitors as one class.
In the second part of the test, the classifier learns the properties of each capacitor, while also withstanding variation within the same class. In this way, it is possible to distinguish different capacitors by their color and markings, even though they look similar visually. Based on the model developed during training, Cognex Deep Learning accurately classifies and aligns capacitors in a single image at runtime.
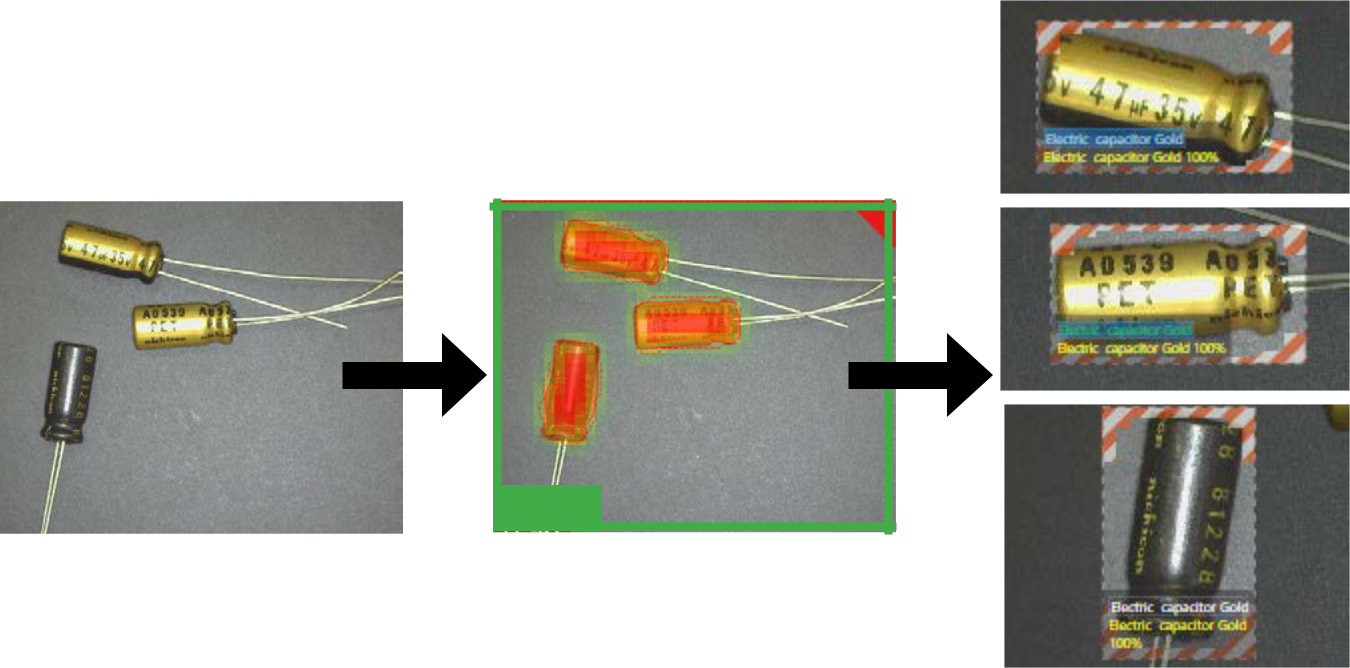
Deep learning tests USB connection
Problem:
The smartphone requires a USB connector for charging and data transfer. This connector is typically an OEM product and should be tested on all sides before being cleared for installation. Connectors include power and data contacts, shields, and some form of mount connectors.
These USB connectors can show various faults, including burning, shorting, dust, scratches and displacement, on all sides. Small, nearly invisible defects on the working end of a connector can greatly affect how well the cable will connect, how tight it will be, and how easy it is to remove. Such a connector must work for the lifetime of the phone.
SOLUTION:
Cognex Deep Learning defect detection engine trains on image datasets that include many functionally important appearance variants and those with no functional impact and learns to distinguish them from each other . During training, it is possible to ignore acceptable cosmetic abnormalities so that training focuses only on defects that may interfere with function, making the process simpler and faster. . When deployed on a line, lighting and part placement are important to capture as many defects as possible.
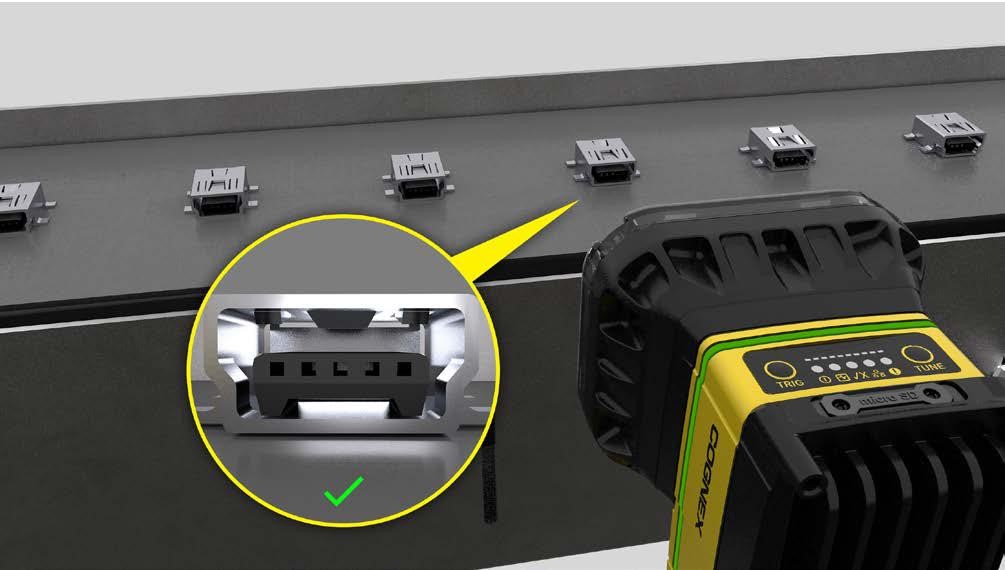
Deep learning OCR testing on PCB assemblies
Problem:
Most chips assembled on a PCB are labeled with an alphanumeric string to keep track of them during manufacturing. Glare can result in low-contrast images, making it difficult for machine vision systems to locate and recognize characters. To successfully decode characters on electronic components and modules, optical character recognition (OCR) systems need to withstand reflective surfaces as well as distorted, misaligned and poorly engraved.
Solution:
With Cognex Deep Learning, you can easily read distorted characters, despite visual difficulties. This deep learning-based OCR approach saves training and development time by reducing over-labeling and successfully reading characters in difficult situations. The software only requires an engineer to set the region of interest and character size. Once set up, the tool’s pre-trained font library decodes characters and reads strings without training. In situations where the characters are very difficult to read, the software can be directly retrained using variant characters.
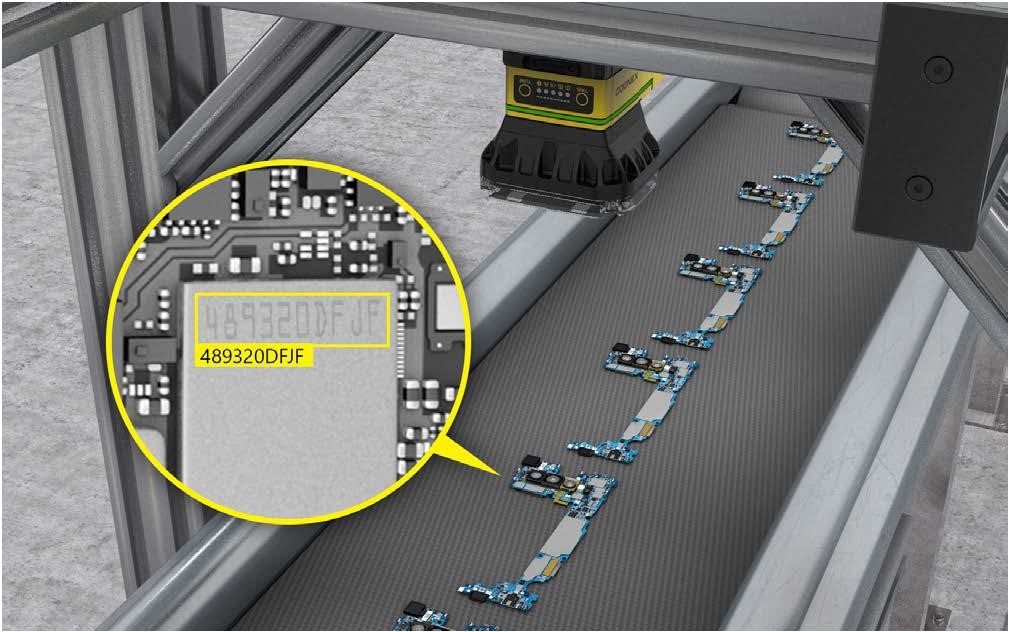
Deep learning to analyze defects
Problem:
After the smartphone is fully assembled but before packaging, the smartphone must be inspected for scratches, cracks, chips, dents, deviations, discoloration and other defects, which may be in many places anywhere on the case and glass. These defects are not generally functional, but negatively affect the appearance of the product.
Traditional rule-based vision applications can be trained on a wide range of typical defects, such as scratches in a predetermined area or cracks that tend to appear in a corner of the screen. , but the range of possible defects is extremely large and can appear anywhere on the phone. Even a relatively infrequent defect, such as a discoloration of the case, or a dent caused by the impact of a robotic arm, needs to be inspected prior to packaging. Given the pace of phone production, human inspection is no match for low efficiency.
SOLUTION:
Cognex Deep Learning trains on sets of images showing a wide range of unacceptable defects as well as sets of images showing aesthetic variations within acceptable ranges and learns to classify them. Parameters can be adjusted during the training phase to ensure that the acceptance and rejection criteria meet market requirements for all examples. Leveraging exceptional lighting and proper presentation combined with Cognex Deep Learning, a trained image analyzer examines the display, strap, back and detects any combination of marks dents, scratches and discoloration anywhere on the smartphone. This thorough and precise inspection ensures that only products that are free of cosmetic defects make it to the packaging step.
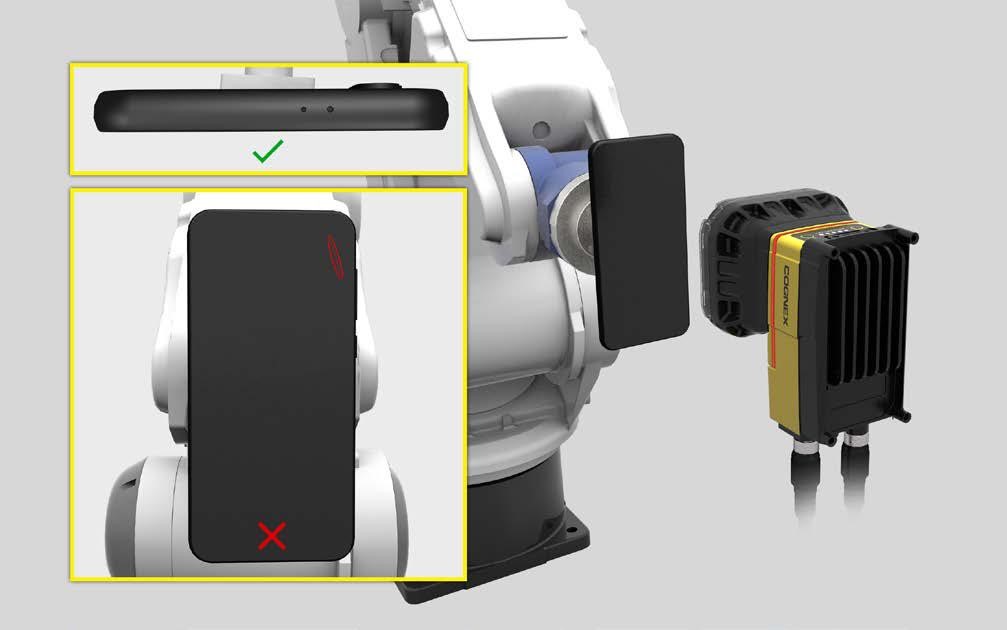
Deep learning tests the filter on the mobile phone's speaker
PROBLEM:
The speaker grille is a perforated piece of metal that protects the mobile phone’s sound module from dust and damage while minimizing the impact on the sound.
Mesh should be visually inspected for cracks, scratches, holes, deformation, broken edges, foreign matter such as dirt or hair, and damage from the punching process. This mesh is visible to the end user, and therefore aesthetic damage must also be detected.
Because meshes are three-dimensional textured parts, they create complex highlights and reflected light patterns. Even a small tilt in the mesh can completely change the model. Meanwhile, defects such as contamination or irregular scratches can appear anywhere on the part. This can be on the outside surface, or in the gaps between the wires. These variations make it difficult for traditional machine vision programming to handle every possible scenario.
SOLUTION:
To identify loudspeaker grille defects, the Cognex Deep Learning defect detector is trained on a wide selection of loudspeaker grilles to learn the full variability of normal parts, including light variations. common light and an acceptable degree of cosmetic defect. When scanning through the meshes, it analyzes and flags any points that fall outside the acceptable range, while minimizing false positives. Cognex Deep Learning can work in conjunction with traditional machine vision, which is best for precise alignment tasks and speaker grid sizing. The combination of deep learning plus traditional vision tools goes beyond manual inspection, with significantly higher speeds. And it does so while maintaining consistency across shifts and production lines.
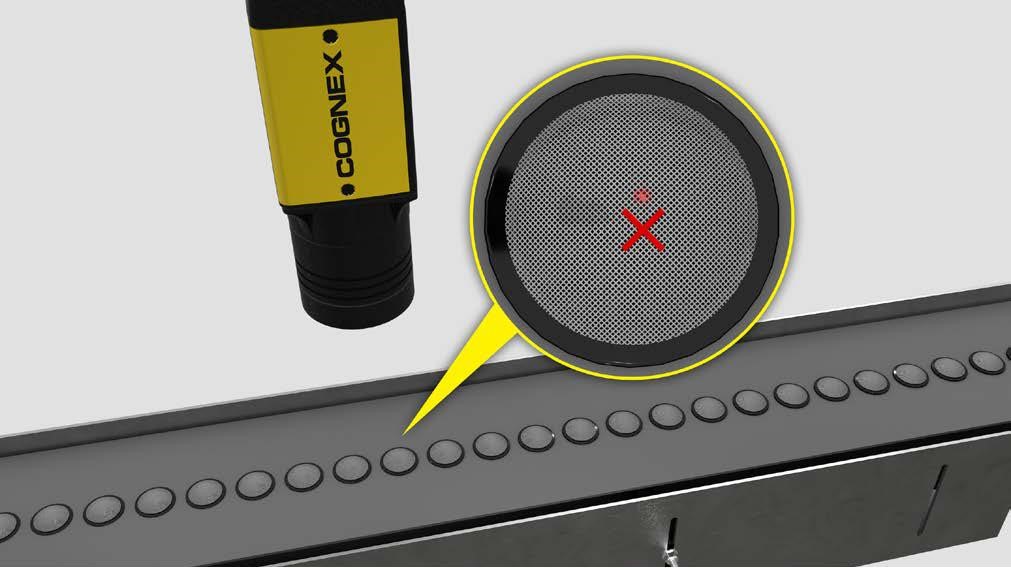
Deep learning checks the surface of the camera module
PROBLEM:
Before installing the camera module to a mobile device, the surface of the module must be inspected to ensure that there are no foreign objects, scratches, stains or dust on the lens.
Different defects are very different in appearance. Fingerprint smudges look quite different from dust particles trapped under the lens coating and also unlike scratches on the glass surface. In addition, the reflective surface of the lens and the refracted image from the elements below the lens may appear as undesirable anomalies even though they are not. Distinguishing these background anomalies from true defects often requires manual inspection, a process that is slow, expensive, and inconsistent. However, traditional rule-based machine vision systems cannot easily be programmed to consistently identify so many defects.
SOLUTION:
Cognex Deep Learning’s flaw detection engine is trained on a wide selection of defect-free lenses to learn the full variation of normal parts. In Unattended mode, it scans through a sequence of lenses and flags any that are out of acceptable range, while minimizing false positives.
Lens defects tend to have a number of characteristics stemming from specific causes, such as contamination by dust and other particles, smudges from oil or fingerprints, and internal components. inside is deviated. Users who need to identify a specific type of defect, or accurately measure the size of a defect, can use the monitoring mode. In this mode, the user trains the system on the combination of good and bad parts, clearly highlights the defect areas and labels them with the defect type: scratch, stain, contamination, etc
This knowledge can be used to control the upstream process. A certain type of scratch can be caused by machine misalignment or yarn can be deposited due to poor airflow during manufacturing. By identifying the root cause of the problem, manufacturers can quickly take corrective action and reduce the production of more bad parts.
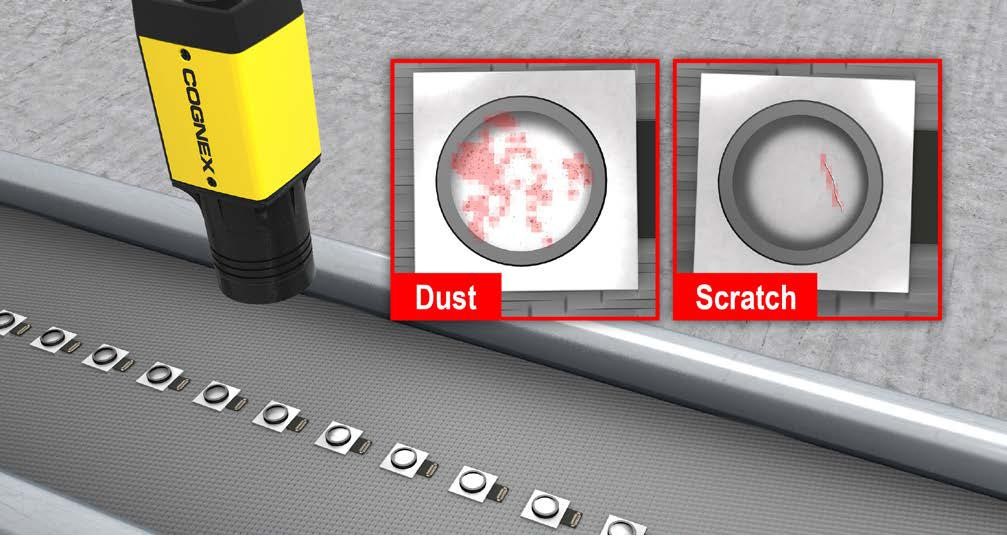
Deep learning checks solder mark on Battery
PROBLEM:
When a battery cell is sealed, it is filled with liquid electrolyte through a small hole in the cap. When filling is complete, the hole is covered with a sealing pin or cover, which is then welded into place to secure it.
The proper position of the sealing pin has traditionally been confirmed by determining the height of the pin with a laser or other 3D displacement sensor. But defects in the weld itself, such as dents, burn marks, pinholes and breaks, are not easily detected through traditional machine vision due to the wide range of possible defects and reflective backgrounds. of the battery cover.
SOLUTION:
Once the weld is complete, the Cognex Deep Learning defect detection tool can identify a variety of potential defects on the seal pin weld. The deep learning application is trained on a variety of properly sealed batteries to learn the full variability of normal parts, including acceptable levels of cosmetic defects, highlights, and shadows.
Once on the production line, Cognex Deep Learning will analyze and flag any dowel welds that fall outside the acceptable range, while minimizing false positives due to purely cosmetic defects.
Users can transfer detected defects to Cognex’s classifier. The tool is trained on a wide range of weld defects and labeled locations so they can be identified properly. At runtime, the classification data is used to take corrective action upstream for any type of defect detected. Depending on customer preference, Cognex DSMax, a laser displacement sensor 3D, can easily be integrated into the inspection process without any third-party software. Together with Cognex Deep Learning and DSMax it is possible to inspect the entire seal and its weld to ensure it is free of defects and is welded and placed correctly.
This knowledge can be used to control the upstream process. A certain type of scratch can be caused by machine misalignment or yarn can be deposited due to poor airflow during manufacturing. By identifying the root cause of the problem, manufacturers can quickly take corrective action and reduce the production of more bad parts.
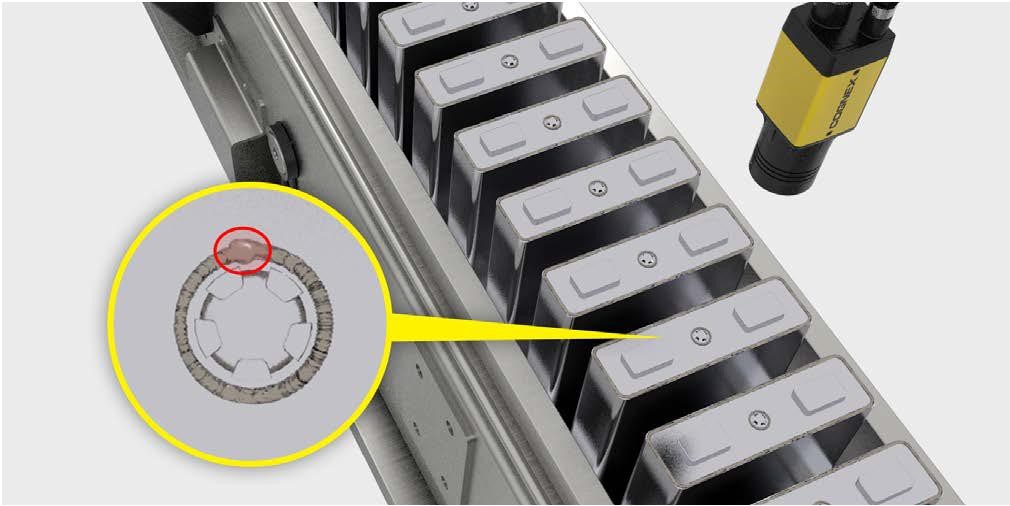
Deep learning checks the battery's label
PROBLEM:
The battery electrodes of the bag connect to external circuits by metal tabs. These foil nodules are made of a variety of metals, are thin and soft, and can be damaged easily. Typical defects include scratches, bumps, holes and dust. Broken tabs reduce or prevent connection, which is why they must be defect-free before being soldered to the electrodes.
However, improper welding also leads to defects such as burns, bumps, lack of balls. Poor solder leads to reduced or missing connections and reduced performance.
SOLUTION:
To identify both tab and tab weld defects, the Cognex Deep Learning defect detection engine is trained on a wide selection of undamaged and properly welded tabs to learn the full variation of normal parts, including an acceptable degree of cosmetic defect.
When scanning through pin tab connections, it analyzes and flags any tabs or tab welds that are out of the acceptable range, while minimizing false positives due to purely functional defects. America. It makes these differences despite contrast and background differences. Cognex Deep Learning’s classifier can then train a bunch of labeled tab and tab solder defects and learn how to classify specific types of defects, such as scratches, holes and bumps on the tab or burn, impact or missing balls on the solder. These classified defects can then be used to control the upstream process to minimize defects over time.
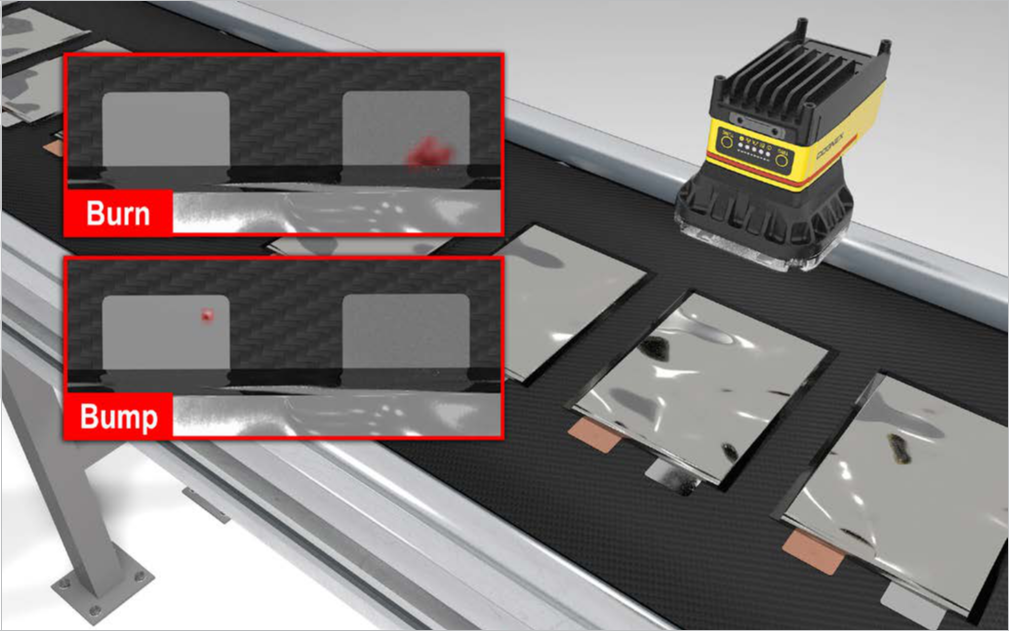
Deep learning tests Pin's button
PROBLEM:
Button (or coin) and cylindrical batteries are sealed by welding the cap to the housing containing the electrode and electrolyte. Low heat methods such as laser or resistance welding are commonly used to avoid damage to the battery inside but require precision to ensure that the cap seal is held tight. There is little margin for error. Defects can lead to electrolyte leakage and reduced performance.
Welding defects can appear anywhere around the perimeter and can vary widely in appearance. Dimples, scratches, and other defects can appear anywhere on the body. Both types of defects can be difficult to distinguish for the reflective surface of the cell. All of this makes it difficult for traditional machine vision to accurately detect all defects.
And because these batteries are manufactured in large quantities, and defects are small and difficult to detect, they pose a similar challenge to manual inspection.
SOLUTION:
To identify battery weld defects, the Cognex Deep Learning defect detector is trained on a wide selection of properly sealed batteries to learn the full variability of normal parts, including including an acceptable degree of cosmetic disability. As it scans through the battery covers, it analyzes and flags any welds that fall outside the acceptable range, while minimizing false positives due to purely cosmetic defects. It’s up to your preference. customer, the Cognex DSMax, a 3D laser displacement sensor, can easily be integrated into the inspection process without the need for any third-party software. Together with Cognex Deep Learning and DSMax it is possible to inspect the entire seal and its weld to ensure it is free of defects and is welded and placed correctly.
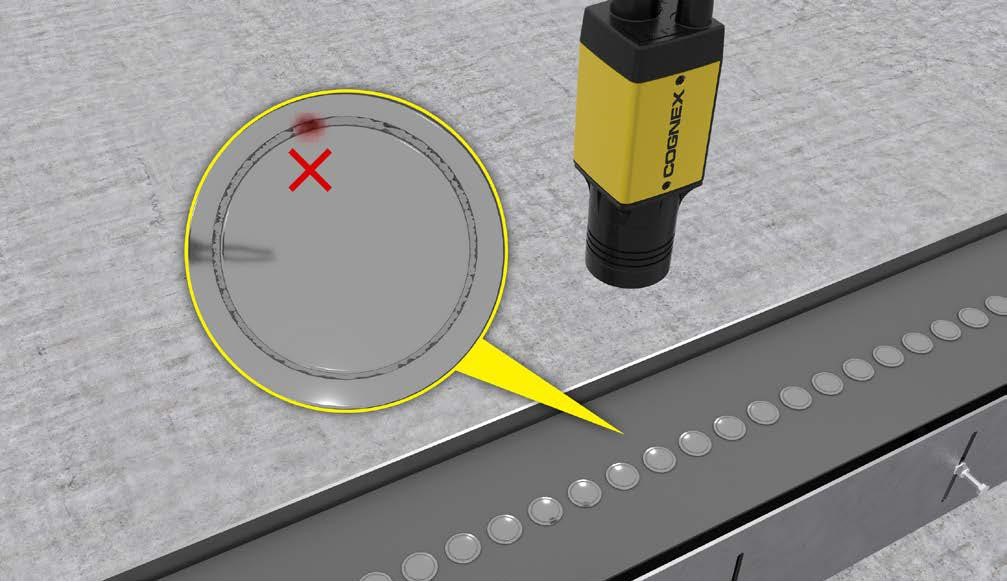
Deep learning checks solder marks
PROBLEM:
To ensure a solid electrical connection, the conductors must be soldered in place to each other or to the terminals. Welding involves melting two dissimilar metals together to form a solid connection. It is important that the weld is sufficiently fused, of sufficient volume but not excessive, of good shape and properly positioned. Manufacturers tend to have multiple lines for many types of electronic parts and need to ensure that all connections are secure.
Because spot welds are highly variable and heterogeneous in appearance, including shape, position, color, reflectivity, texture, and surface markings, inspection can be high rate of false positives, also known as overkill. Overkill leads to good parts being discarded. Good welds that are incorrectly marked as defective require manual inspection, which is extremely slow compared to line speed, and still often results in incorrectly identified weld defects.
Parts also vary in size, color and other characteristics from batch to batch. The wide range of variations and difficulty distinguishing good from bad connections make rule-based machine vision impractical.
SOLUTION:
Users train Cognex Deep Learning’s fault detection engine on a wide selection of appropriate spot-welded connections to learn the full variation of normal parts. As the tool scans through the welds, it analyzes and flags any points that fall outside the acceptable range, while minimizing false positives. Cognex Deep Learning’s classifier can then Train in a wide range of labeled welding defects and learn to classify specific types of defects, such as poor shape, blowholes, cracks, burns or surface contamination. These classified defects can then be used to control the upstream process to minimize defects over time.
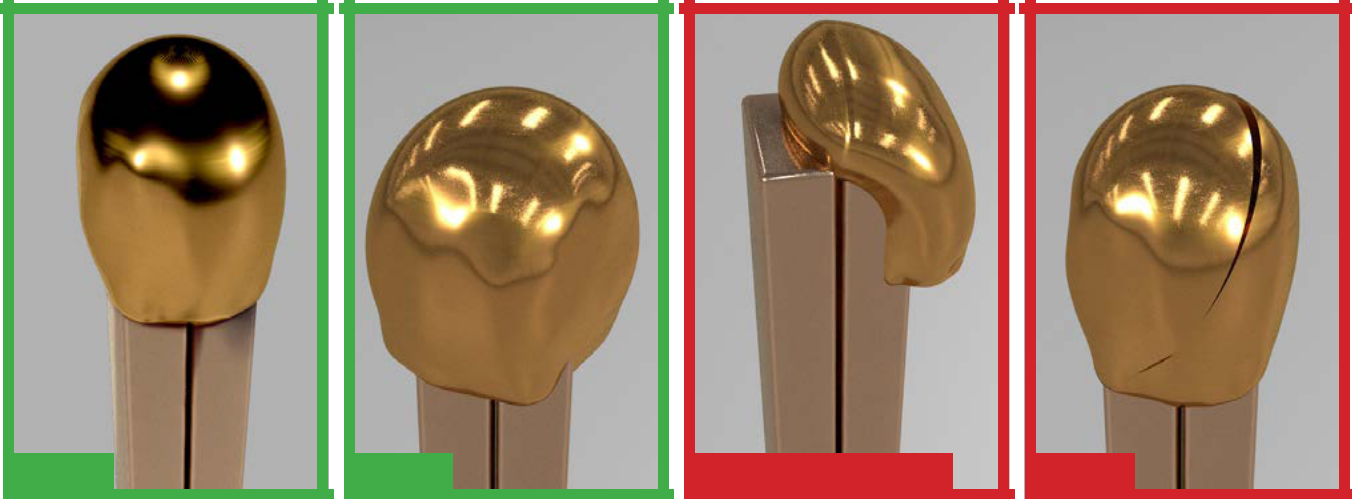
Deep learning tests MLCC
PROBLEM:
Multilayer Ceramic Capacitors (MLCC) consists of a stack of capacitors with metal plated connectors for connection to integrated circuit boards. MLCCs suffer from many possible manufacturing defects, including cracks, blisters, crumbs, contamination, and gaps in the finish coat. These capacitors store significant energy, so failure not only affects the faulty MLCC but can damage neighboring components or the integrated circuit board itself.
MLCCs are small and large in number. They can have a variety of minor defects that vary in shape and location. In addition, they have a shiny surface that limits the effectiveness of traditional machine vision.
Therefore, manual testing still plays an important role. Automated optical testers (AOI) test all six sides of all capacitors, then human checks one side of the statistical sample of the capacitors. But AOI machines have a high overkill rate, while manual testing is too slow for general use. The overall process is expensive, slow, error-prone, and doesn’t produce useful data that can help improve the process.
SOLUTION:
Cognex built a special aesthetic optical inspection (COI) machine for MLCC inspection, combining both custom lighting and deep learning vision tools. First, the lighting module is customized for MLCC testing minimizing extraneous surface variations while revealing easily missed defects on both the capacitor body and terminals.
After the MLCCs have been tested with an AOI machine, they are tested with a COI machine to reduce the amount of false positives and good parts being pulled out of the manufacturing process. This machine delivers better speed, accuracy and process improvement data when compared to manual inspection.
Cognex Deep Learning’s classifier is trained on labeled images of a variety of defect-free and defect-free MLCCs. The classifier learns to classify the wide range of possible defects, as well as learns the full variability of normal parts. Once trained, it can scan through any MLCC parts and immediately flag any parts that fall outside the acceptable range or identify good parts that were previously flagged as defective.
Classified defects can also be used for upstream process control to minimize part defects over time.
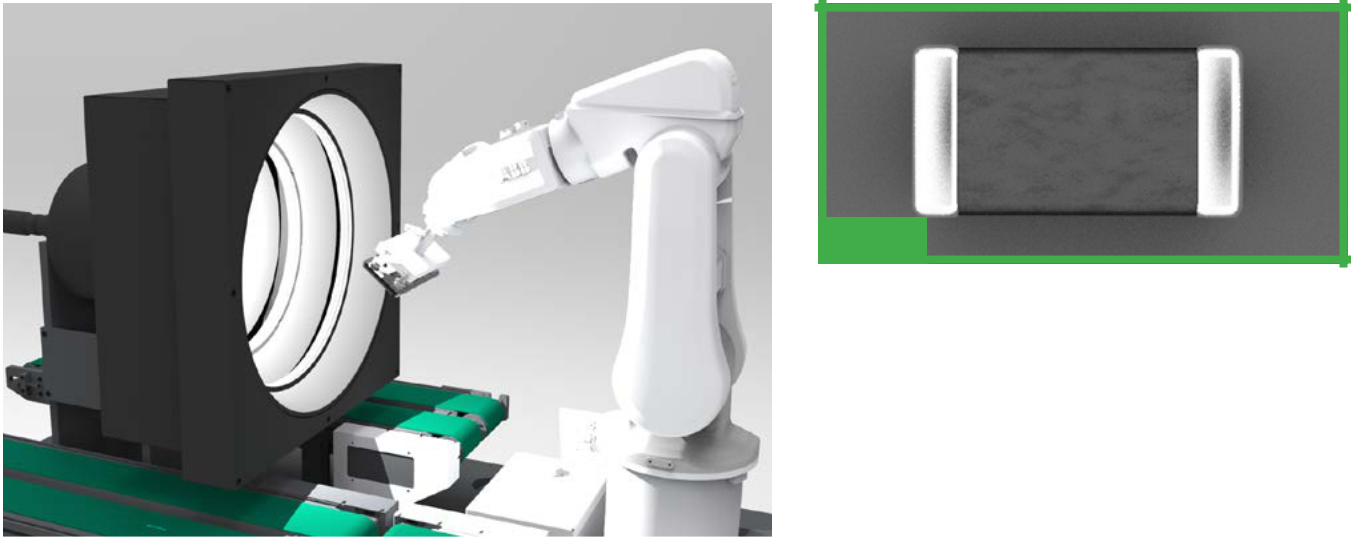
DEEP LEARNING SOLUTIONS
Cognex Deep Learning is the first set of deep learning-based vision solutions specifically designed for factory automation. Proven, optimized, and field-proven technology based on state-of-the-art machine learning algorithms.
Instead of following a rule-based approach to solving inspection challenges, like traditional machine vision applications, Cognex’s deep learning solutions learn to detect patterns and anomalies from objects. Reference image example. Deep learning automates and scales complex testing applications that hitherto still required testers such as defect detection and final assembly verification.
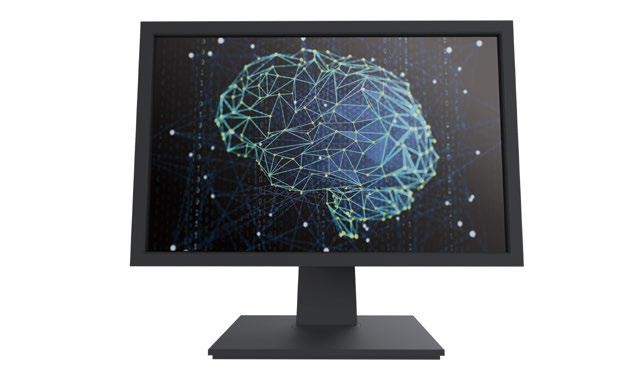
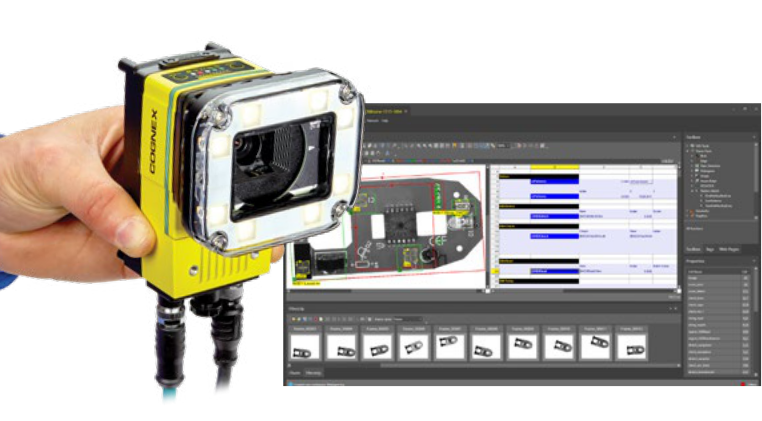
In-Sight ViDi
Deep learning applications In-Sight® ViDi implemented on smart camera In-Sight D900 without a PC, making it easy for non-programmers to access deep learning technology. The application uses the familiar and easy-to-use In-Sight software platform, which simplifies application development and factory integration.
VisionPro Deep Learning
Software VisionPro Deep Learning combined machine vision tool library (Machine Vision) Comprehensive with advanced deep learning tools within a common development and deployment framework. It simplifies the development of highly scalable vision applications and enables engineers to build flexible, highly customized deep learning solutions tailored to specific needs.
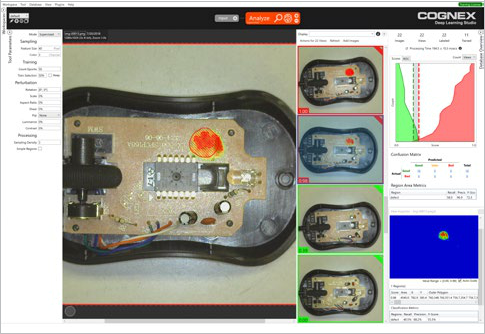