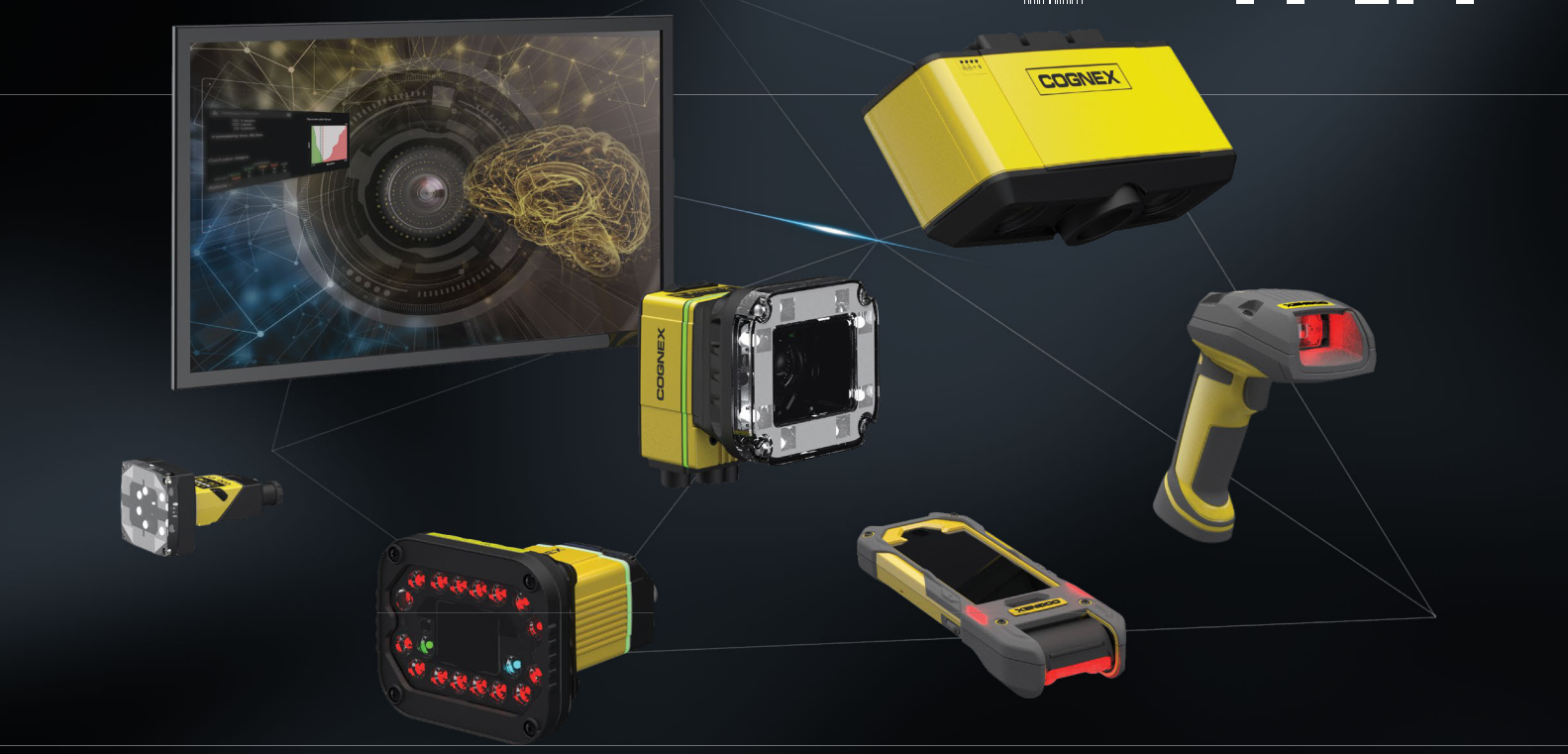
DEEP LEARNING SOLUTION FOR ASSEMBLY VERIFICATION
Today’s machine vision algorithms can distinguish parts by even the slightest difference in markings or features. However, limitations still exist, so it takes programming to develop rules for hundreds or even thousands of variations where a single part can appear to the camera. Even with the best programmed rules, the very complex scenes involved in assembly verification applications can be too difficult to control. In particular, partial changes, large numbers of components, and configuration changes are difficult and time-consuming to program using traditional machine vision. Deep learning vision software provides a groundbreaking method for automating this layer of testing. Cognex Deep Learning Solutions can be trained to handle a wide range of partial changes to determine and confirm whether components are present and correct. The application can also recognize many types of components in different layouts and configurations.
Verification of car assembly at the final stage
Ensure all car components are present and correctly assembled using deep learning software
Problem:
Machine vision is used throughout the automotive component manufacturing process to rigorously monitor and detect quality defects. Final assembly verification is often done with the operator, as the different pieces involved in final assembly create a high level of complexity that makes traditional machine vision inspection difficult. The tester verifies that all components, such as interior components such as door trims, window switches, and door handles, are present and assembled correctly. Exterior testing of colors, badges, headlights and other components is also carried out at the final stage of car assembly. Inspection personnel, while skilled at identifying different parts as different models move down the line in changing lighting conditions, can be slow and inconsistent.
Solution:
Cognex Deep Learning looks at the finished appearance of many car parts to identify improperly placed parts. The app can do this exactly like a tester, but with the speed and reliability of an automated system. Using the assembly and part location verification tool, users can build a component library of trained features.
This component library can contain a wide range of parts, from badges to door handles, to locating and identifying tools in images. By adding a verification step, the software can provide pass or fail results based on all components that must be assembled. Using this approach, final assembly verification can now be automated.
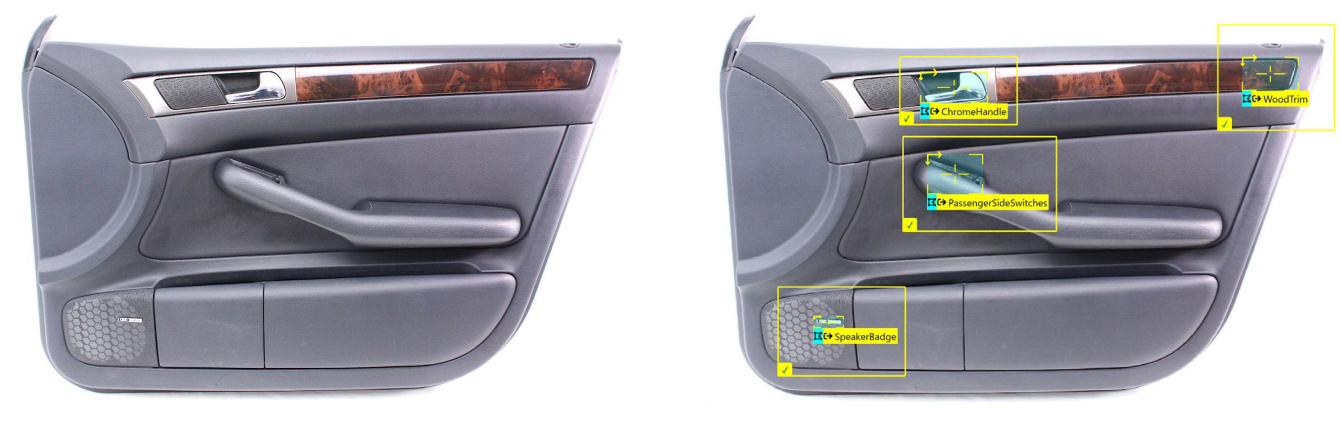
Check the motherboard
Identify and count PCB components to ensure correct assembly using deep learning software.
Problem:
During final assembly verification, 2D and 3D machine vision systems inspect the PCB for the presence and correct location of LEDs, microprocessors, and other surface mount devices. Missing or misplaced components can affect the performance and life of the PCB. Manufacturers must be wary of pins that are scratched, twisted, bent, or missing. A chip has such low fault tolerance that any defect, even the most superficial, is cause for rejection.
These defects must be detected before the PCB is assembled into the device or shipped to the customer. However, small changes in appearance — whether due to subtle light contrasts, changes in viewing angle and direction, or glare on metal surfaces — can confuse automated inspection systems. . Parts that are close together are difficult for the machine vision system to distinguish as independent parts. Programming these checks into a rule-based algorithm is time consuming, error prone, and challenging for a field engineer to maintain. Test personnel, despite being able to identify these components, cannot meet the demands of high-speed processing.
Solution:
Cognex Deep Learning provides a field-maintainable solution for PCB assembly inspection. Assembly Verifier learns to identify components from images labeled with the location of each part type, building a reference model of each component in a single tool. This tool generalizes the distinguishing characteristics of parts based on their size, shape, and surface features and learns their normal appearance, as well as their general position on the board. The system is also optimized to work with low-contrast or poorly captured images.
In manufacturing, all tools are in the area involved to locate and identify each component, regardless of changes in appearance. This solution can determine if the component is present or correct and verify that the component is being used. In this way, the tool can provide a reliable solution for automating complex PCB assembly inspections.
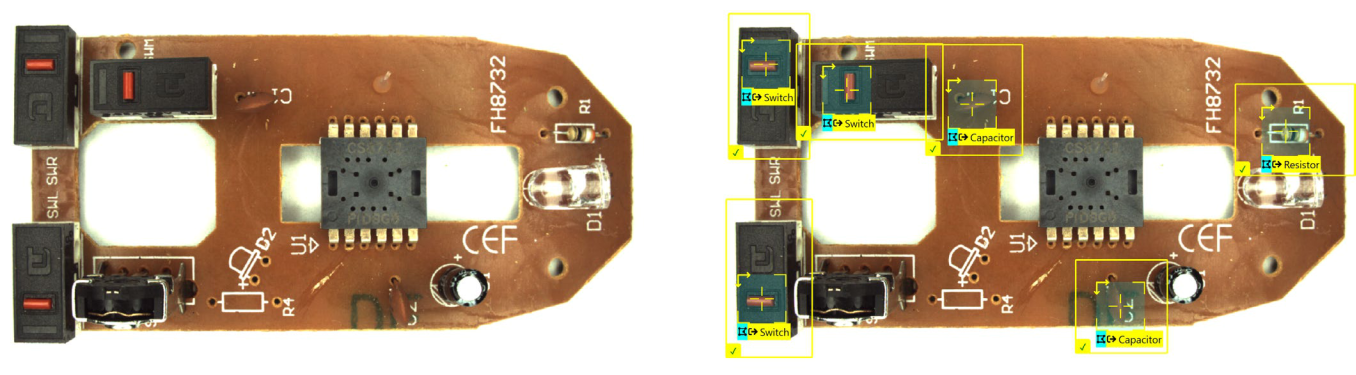
Chocolate test
Identify, count and verify chocolates based on their appearance to verify packaging and assembly using deep learning software
Problem:
For certain food packaging applications, manufacturers must rely on visual inspection to ensure the quality of the final assembly. Visual variations of food products can cause complications for automated food and beverage inspection systems. This is the case with chocolates, packaged in boxes of different configurations. The test system must successfully verify that each spot contains a piece of chocolate and that it is of the correct type.
Holiday-themed chocolate boxes (i.e. Christmas or Valentine’s Day) offer a specific difficulty. The same chocolates may be in the box, but their location may vary depending on the theme of the box. The manufacturer must locate each chocolate and verify the correct one is in the correct location. Similarly, two boxes of chocolate can use the same packaging (i.e. box of 6 chocolates) but have different flavors (e.g. milk and dark chocolate options). In this case, the manufacturer needs to count the chocolates and verify the correct chocolate selection.
Solution:
Cognex Deep Learning automates the task of locating and identifying multiple features in a single image. Deep learning technology can generalize the distinguishing characteristics of different types of chocolate based on their size, shape, and surface features. With the assembly verification tool, users can train the tool to determine where each chocolate will need to be found. Users can build a database of different chocolates for search engines, which can then be used to verify packaging.
Once trained, the image can be divided into different zones where the tool will check for the presence of chocolate as well as verify that it is of the correct type. Multiple configurations can also be created for cases where a single line can have variations in packaging. In this way, users can automate the verification of chocolate packaging with just one tool
Check the metal frame
The defect detection engine confirms the presence and location of components on a cryptic background
Problem:
The various cuts involved in the final assembly verification process create a high level of complexity that challenges traditional machine vision inspection. The tester verifies that all parts, such as the band and metal case, are present and assembled correctly. Subtle light variations make it possible to discern whether the bands are in their correct places. Test personnel, although skilled at identifying bands, can be slow and inconsistent. Cognex Deep Learning analyzes the finished look of a trim and identifies missing bands as accurately as a human tester, but with the speed and reliability of an automated system.
Solution:
Using defect detection, a technician trains the system on a “bad” image of the cut where there is no wire, as well as a known “good” image where there is a wire, to create a Reference model for a complete part. Using this model, deep learning identifies missing wire cutouts as irregular and faulty, damaging them during final inspection.
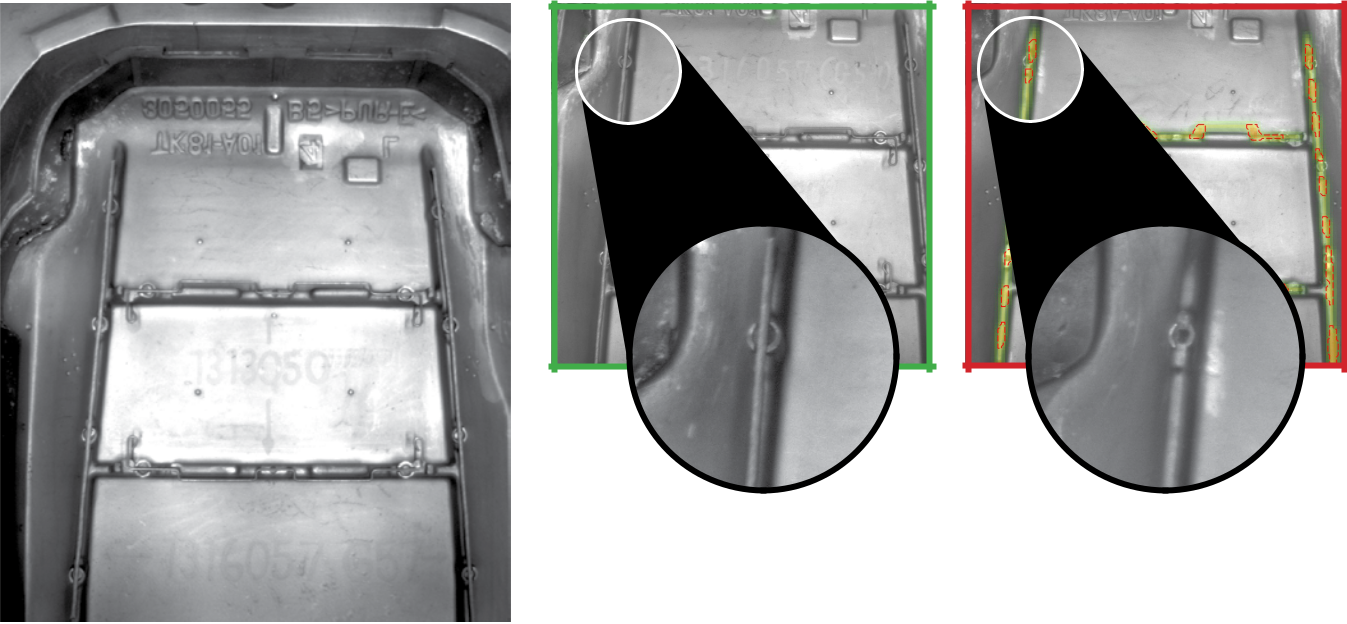
DEEP LEARNING SOLUTIONS
Cognex Deep Learning is the first set of deep learning-based vision solutions specifically designed for factory automation. Proven, optimized, and field-proven technology based on state-of-the-art machine learning algorithms.
Instead of following a rule-based approach to solving inspection challenges, like traditional machine vision applications, Cognex’s deep learning solutions learn to detect patterns and anomalies from objects. Reference image example. Deep learning automates and scales complex testing applications that hitherto still required testers such as defect detection and final assembly verification.
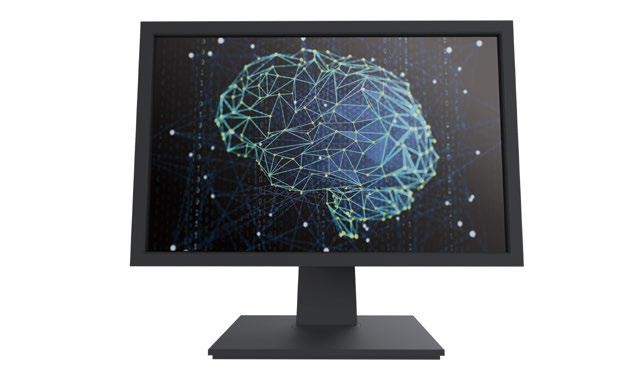
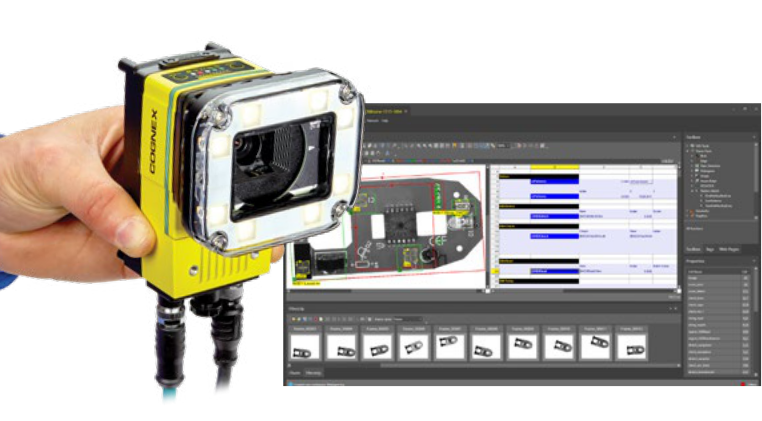
In-Sight ViDi
Deep learning applications In-Sight® ViDi implemented on smart camera In-Sight D900 without a PC, making it easy for non-programmers to access deep learning technology. The application uses the familiar and easy-to-use In-Sight software platform, which simplifies application development and factory integration.
VisionPro Deep Learning
Software VisionPro Deep Learning combined machine vision tool library (Machine Vision) Comprehensive with advanced deep learning tools within a common development and deployment framework. It simplifies the development of highly scalable vision applications and enables engineers to build flexible, highly customized deep learning solutions tailored to specific needs.
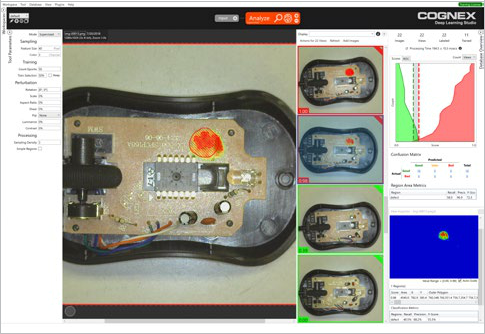