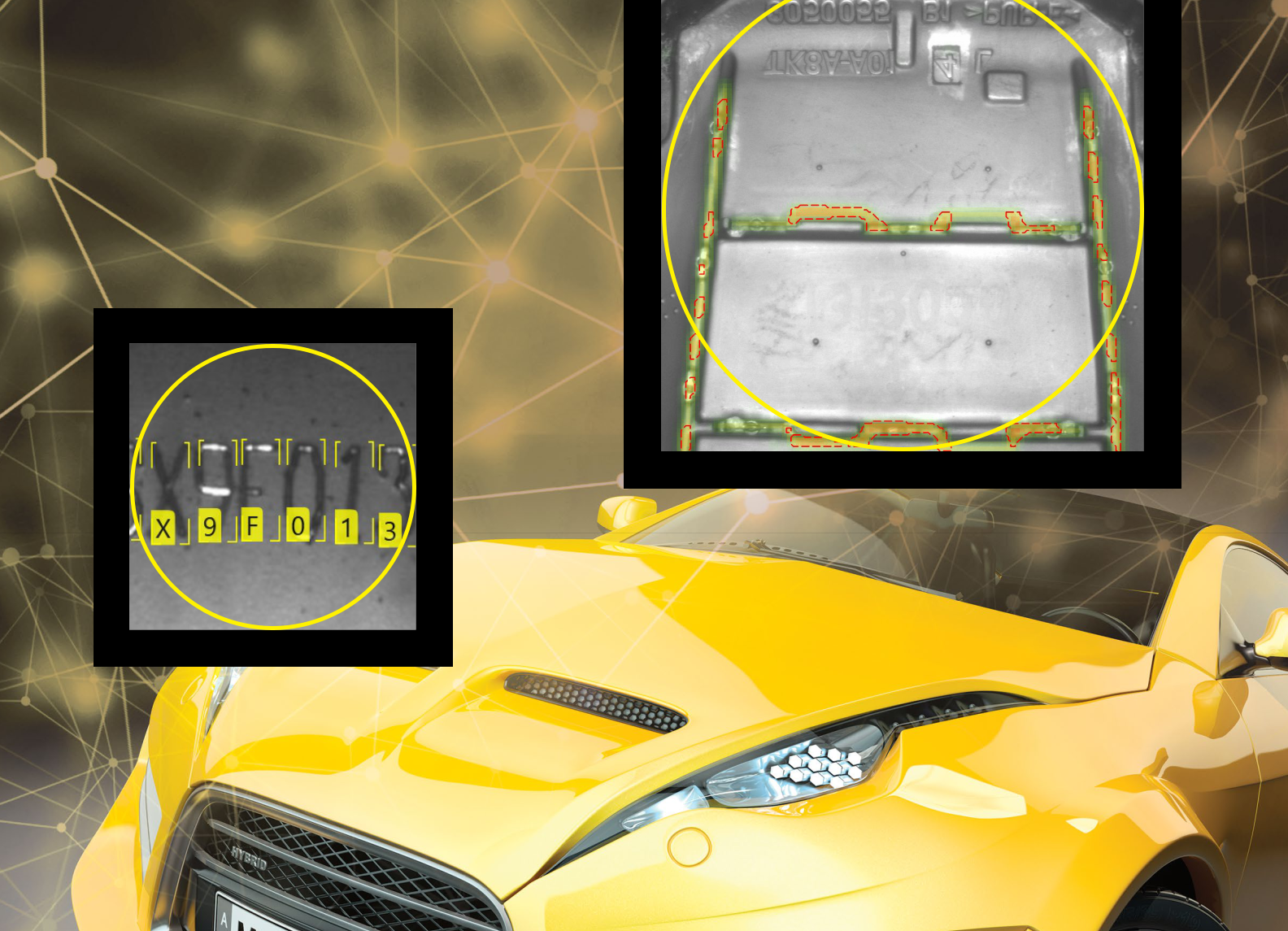
DEEP LEARNING SOLUTIONS IN THE AUTOMOBILE INDUSTRY
Cognex Deep Learning is the first deep learning-based image analysis software designed specifically for the automotive industry. Combining human-like intelligence with the power of machine vision, Cognex Deep Learning is ideal for distorted part placement, inspection, complex cosmetic grading, and full OCR applications challenge. Automate and solve previously difficult programming challenges in an easy-to-deploy interface.
Deep learning application checks piston rings
The defect detection tool simplifies the automatic detection and characterization of structural defects on metal surfaces.
PROBLEM:
The piston’s compression rings serve several functions in reciprocating engines, sealing the combustion chamber and regulating oil consumption. Defects on the compression ring are difficult to detect due to the reflective metal surface of the piston. The cylindrical shape of the piston sometimes makes the image blurry and out of focus. Normal variations in metal’s surface texture are expected as part of the manufacturing process and some—including rust spots, white areas, and even cracks and fissures. on the surface – are allowed to pass the test. However, long scratches that affect piston performance and threaten the compression level in the cylinder are signs of real defects. The test system must be able to withstand normal variations and insignificant anomalies in the surface of the compression ring while identifying any long scratches.
SOLUTION:
Programming this complexity check into a rule-based algorithm would require complex error libraries. Human checking, although more flexible, would be too slow. Cognex Deep Learning provides an efficient testing solution that combines the human ability to evaluate small changes with the reliability, consistency, and speed of automated systems.
Using a supervised mode fault detection engine, an engineer trains deep learning-based software on a representative set of known “good” and “bad” compression ring images. A technician captions known “bad” images for long scratches and “good” images with normal variations and acceptable defects, including rust and cracks. small. Based on these images, the app learns the natural form and surface texture of the piston, as well as the normal appearance of scratches. Additional images can be added to the training set during validation to reflect additional examples and help with system optimization. The parameters can be continuously adjusted during the training and validation phase until the trained model correctly detects and segments all the long scratched images.
At runtime, the software that described long scratched images as faulty, learned to recognize and ignore unrelated variations.
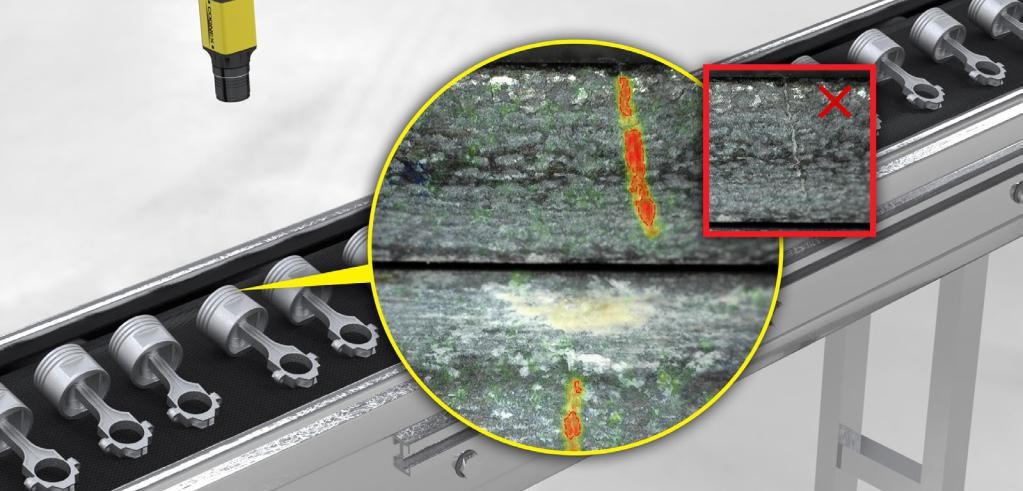
Deep learning cylinder test
The flaw detector is the void in the metal.
PROBLEM:
The cylinder block is the foundation of an automobile engine. Its large cylinders are the main working part of a reciprocating engine, built to hold the pistons as they pump up and down under compression. Cylinders are usually made of cast metal and are sometimes lined or “hand-wrapped” in a lubricating coating. Cylinder walls must be durable because they are in contact with the piston’s compression rings.
The cylinder should be able to withstand minor irregularities in its metal, smoothed during mechanical wear, but shall not contain bubbles in the metal known as “pores”. These defects are especially difficult to check because the surface of the cylinder is rough and the image appears blurry around the edges due to the depth of field. Characteristic light or glare on the reflective surface of the cylinder also complicates inspection. It is very difficult to program automated tests that can withstand so many small changes in feature shape and position, as well as luminance and opacity.
SOLUTION:
Cognex Deep Learning quickly identifies pores when other methods attempt to test under the same lighting conditions. Within minutes, an engineer can train the software on a representative set of “good” and “bad” images of a cylinder, adjusting the region of interest with a masking filter to remove the bright disc of the cylinder. negative space in the axis. Using a defect detection tool in supervised mode, the technician annotated the pores in the image labeled as “bad” and adjusted parameters, including feature size, scale and scale. , aspect ratio, and cropping to help the model interpret changes in appearance. A “Good” image depicting normal cylinders helps the software learn what types of irregularities and small molding variations are acceptable. Engineers can retrain the system, adjust parameters, and add additional images, until the model can generalize to the normal shape of a cylinder and recognize abnormalities. At runtime, deep learning-based software examines each image within milliseconds, identifying those with pores as defective and the rest as normal.
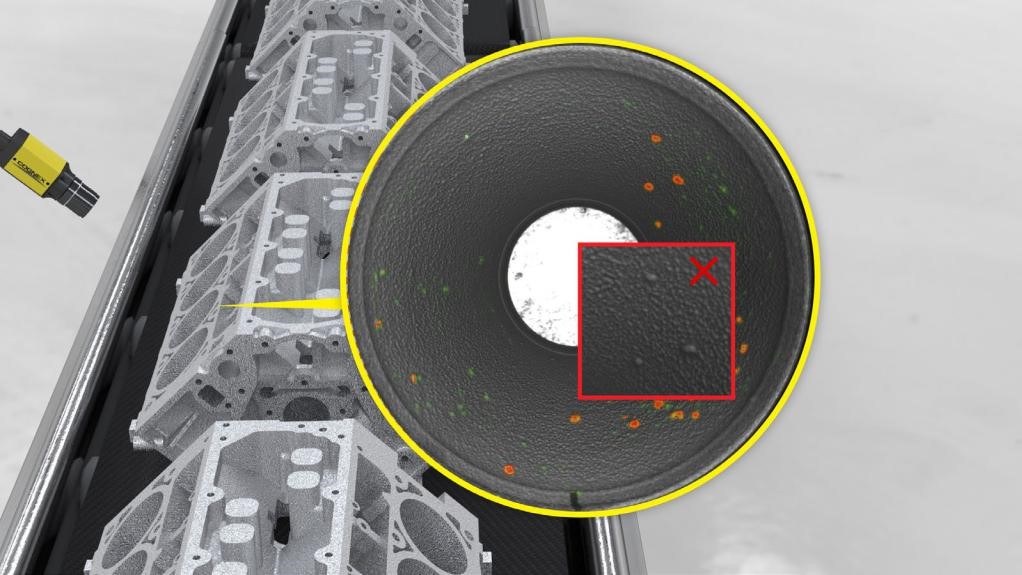
Deep learning weld inspection
Defect detection and classification tools simplify inspection and automatic classification of weld defects
PROBLEM:
Cognex Deep Learning checks the integrity of critical powertrain components such as pistons, whose surface textures make traditional machine vision inspection difficult. Piston welds are highly variable, making irregularities difficult to distinguish. It is not desirable to have some anomalies when welding, such as under-weld, over-strength or poor-quality welds. Other anomalies, such as overlapping seams, are desirable and necessary for safety reasons. Dark image areas introduce additional complications. With many possible errors and lighting challenges, deep learning-based analytics provides a simple and powerful alternative to traditional machine vision inspection.
SOLUTION:
With Cognex Deep Learning, automatic analysis of metal piston welds is simple. Software engineer with supervised mode defect detection on a set of “bad” images representing all welding abnormalities, including overlapping seams and over the “good” samples did not have any anomalies. In this way, all anomalies — both desirable as well as those that cause rejection — are identified as defective.
In the second part of the inspection, the engineer uses the classifier to classify seam defects by type. Based on the model developed during monitoring, the software extracts information about specific defects and separates overlapping seams into their own layer. By using these tools together, the automaker can rest assured that the inspection system identifies all welds and successfully classifies overlapping seams. Based on this information, the manufacturer can choose only overlapping seams to use.
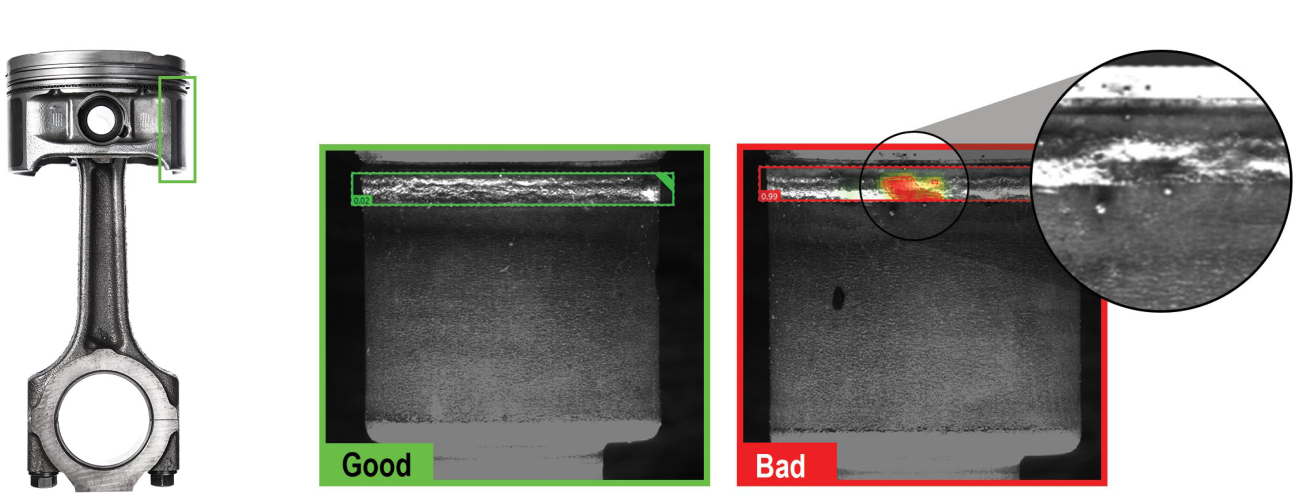
Deep learning igniter identification and classification (spark plugs)
Assembly verification and classification tools identify, count, and classify parts based on their appearance for assembly/pre-assembly verification
PROBLEM:
For certain identification, counting and sorting applications, manufacturers must rely on visual inspection when their environment does not support barcode reading technology. Small changes in appearance can cause complications for automated testing systems. This is the case for spark plugs, which are pre-assembled on trays of different colors. The test system must successfully identify, count and classify spark plugs of different colors while ignoring the background color of their trays. This information is then transmitted to a vision-guided robot for assembly.
SOLUTION:
Cognex Deep Learning generalizes the distinguishing characteristics of a spark plug based on its size, shape, and surface features. With the assembly verification tool, an engineer arranges a tray image, teaching the software to identify and count each spark plug. The classifier uses deep learning-based modeling to classify spark plugs by their robot-matching characteristic — its color.
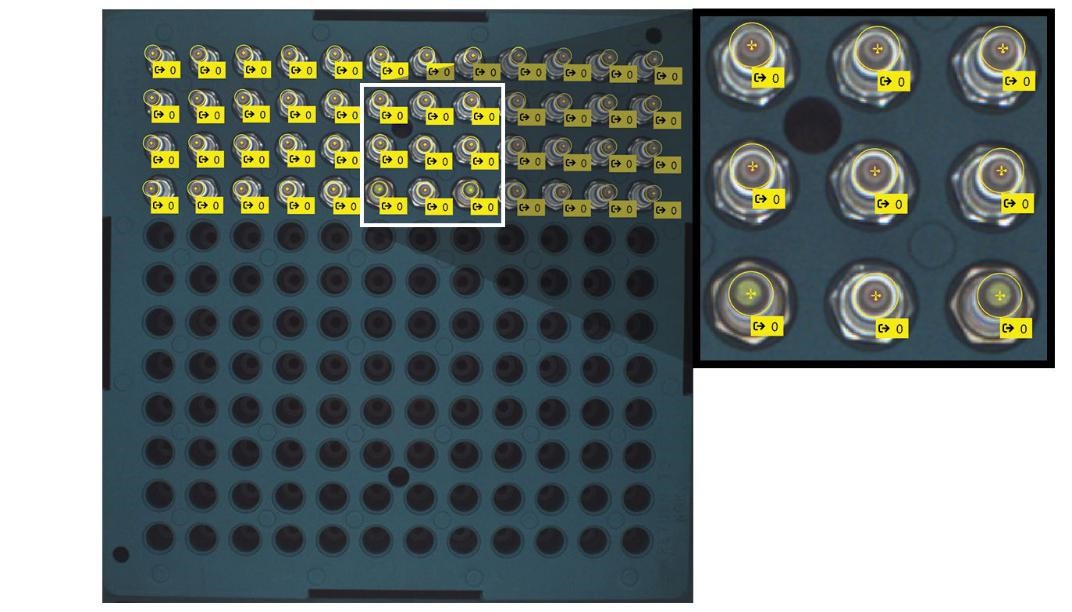
Deep learning to check the airbag
Tool to check for aesthetic defects of textiles
PROBLEM:
Airbags follow strict quality standards to ensure passenger safety. Automakers must double and triple test all safety-critical components to ensure quality, reduce warranty costs, and reduce recall liability. This is especially important for airbags, which must be checked for punctures, rips, rips and seam problems that could cause them to fail. These types of quality issues are often missed or difficult to detect during manual testing. They are also difficult to program into traditional machine vision systems because of the complex woven surface of the airbag. Fabric patterns can be very complex, and the visual appearance between air pockets varies dramatically due to the stretchy nature of the fabric, yarn thickness, and countless small acceptable variations. Since clearly finding all defects is too complex and time consuming, Cognex Deep Learning provides a simple solution to identify all anomalies without training on “bad” images. “.
SOLUTION:
An engineer uses an unattended-mode fault detection tool to train the software on a set of “good” airbag images to build a reference model of the airbag. The model explores the normal appearance of the airbag fabric, including the weave, fabric properties, and color. All features other than the normal appearance of the model are considered abnormal. In this way, Cognex Deep Learning reliably and consistently detects all anomalies, such as holes, rips, rips, and unusual stitch patterns. Faulty areas of fabric can be quickly identified and reported without the need for an extensive error library.
An engineer uses an unattended-mode fault detection tool to train the software on a set of “good” airbag images to build a reference model of the airbag. The model explores the normal appearance of the airbag fabric, including the weave, fabric properties, and color. All features other than the normal appearance of the model are considered abnormal. In this way, Cognex Deep Learning reliably and consistently detects all anomalies, such as holes, rips, rips, and unusual stitch patterns. Faulty areas of fabric can be quickly identified and reported without the need for an extensive error library.
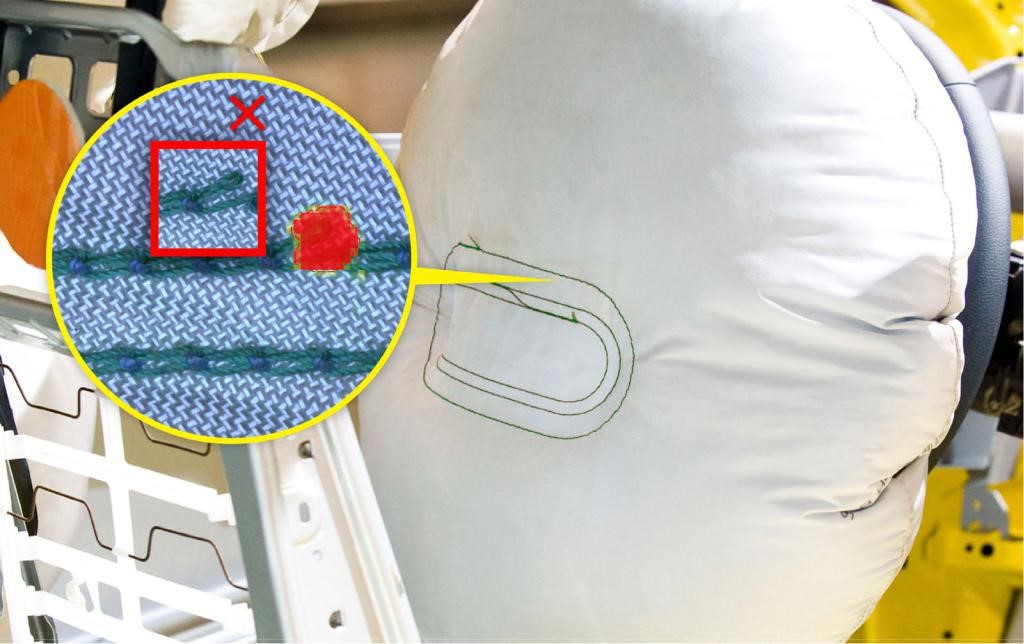
Deep learning final validation for metal frames
The defect detection tool confirms the presence and location of components on hard-to-see background
PROBLEM:
The various cuts involved in the final assembly verification process create a high level of complexity that challenges traditional machine vision inspection. The tester verifies that all parts, such as the band and metal case, are present and assembled correctly. Subtle light contrast makes it easy to tell whether the bands are in their right places. Inspection personnel, although skilled at identifying wire strips, were slow and inefficient. Cognex Deep Learning uses deep learning-based image analysis to learn the finished look of a decorative piece and identify missing bands as accurately as a human examiner, but with speed and precision reliability of an automated system.
SOLUTION:
Using a defect detector in supervised mode, the technician trains the system on a “bad” image of the cut where there is no wire, as well as a known “good” image where the wire is wired. , to create a reference model for a complete piece of decoration. Using this model, the application identifies decorative pieces with missing wire strips as irregular and defective, damaging them during final inspection.
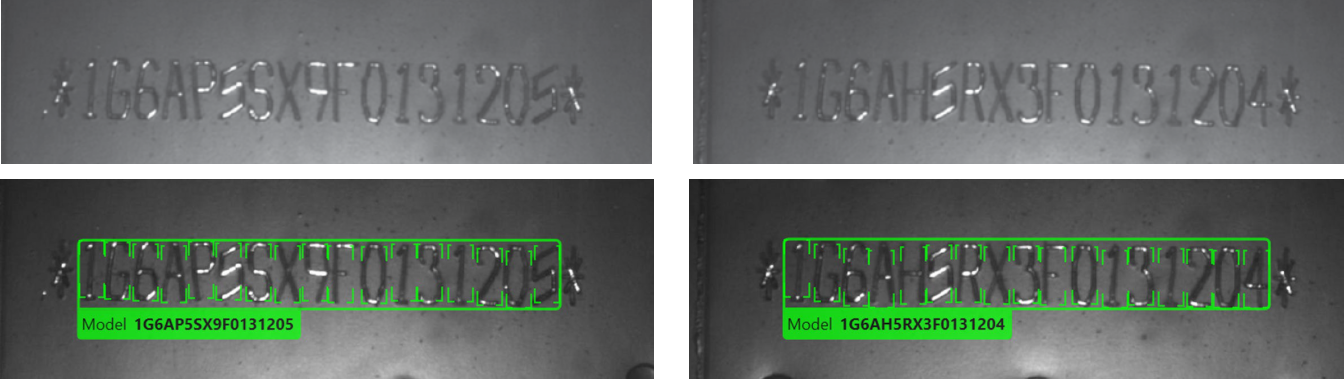
DEEP LEARNING SOLUTIONS
Cognex Deep Learning is the first set of deep learning-based vision solutions specifically designed for factory automation. Proven, optimized, and field-proven technology based on state-of-the-art machine learning algorithms.
Instead of following a rule-based approach to solving inspection challenges, like traditional machine vision applications, Cognex’s deep learning solutions learn to detect patterns and anomalies from objects. Reference image example. Deep learning automates and scales complex testing applications that hitherto still required testers such as defect detection and final assembly verification.
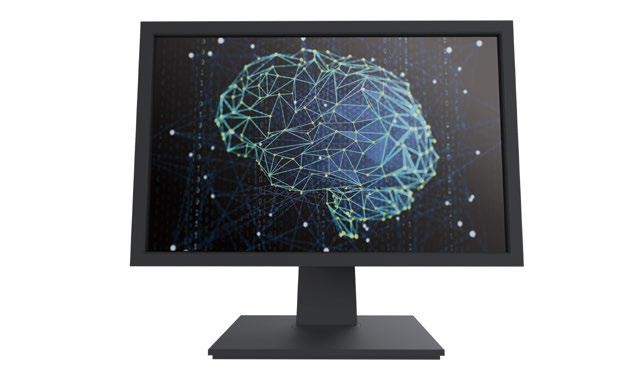
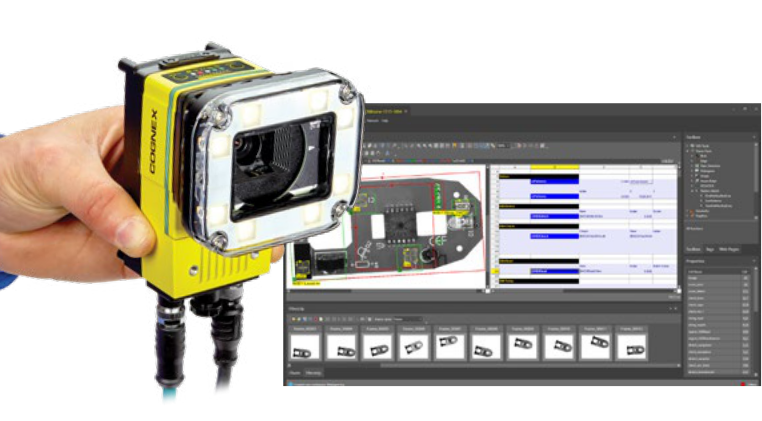
In-Sight ViDi
Deep learning applications In-Sight® ViDi implemented on smart camera In-Sight D900 without a PC, making it easy for non-programmers to access deep learning technology. The application uses the familiar and easy-to-use In-Sight software platform, which simplifies application development and factory integration.
VisionPro Deep Learning
Software VisionPro Deep Learning combined machine vision tool library (Machine Vision) Comprehensive with advanced deep learning tools within a common development and deployment framework. It simplifies the development of highly scalable vision applications and enables engineers to build flexible, highly customized deep learning solutions tailored to specific needs.
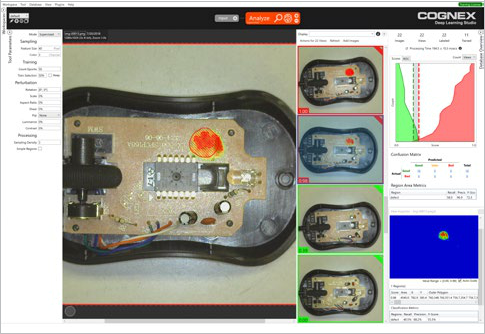